In the life of a business, management usually introduces innovations aimed at improving revenue. Sometimes they get it right; at other times, it results in failure.
In 2011, Apple
’s successful senior vice president Ron Johnson joined J. C. Penney as the CEO.
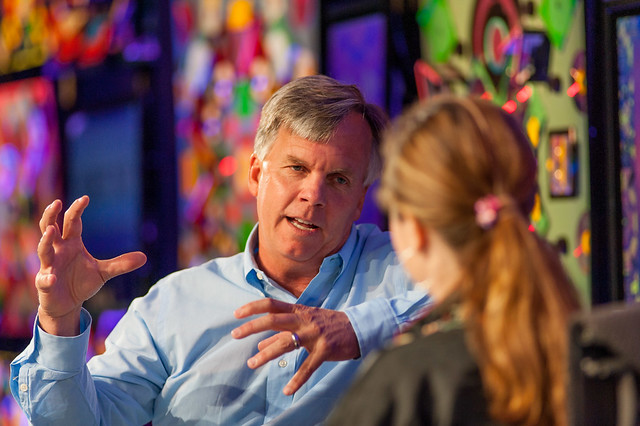
Without any testing, he immediately introduced a change that decimated Penney’s revenue. He introduced technology, which took over the cashiers’ duties. This made the checkout experience too formal. All forms of clearance sales and discounts stopped. Also, high-priced branded products became prominent in the stores.
It took only a year and five months after these changes for J. C. Penney’s sales revenue to crash to an all-time low.
Management reacted. They relieved Johnson of his position as CEO and went ahead to reverse the changes.
With all the available data about transaction trends and consumer behavior, how could J. C. Penney not get it right?
Managers often do not have a comprehensive set of data to take the right decisions on innovations. Available data are usually limited to past consumer behaviors.
There is no sign of how these data will behave towards future radical changes. So, without any solid guide, managers rely on their own previous experiences and intuitions.
Yet, the attempt to introduce new angles to a business should not be a hit or miss affair. Many issues are at stake here. Besides corporate will,
consumer behavior
and
feedback analysis are crucial to a successful innovation
.
Even with obvious costumer disaffection, Johnson refused to accept responsibility for the negative reactions. He rather believed that the consumers needed some education to accept the changes.Business experiment encompasses all tests, data collection, and analysis for a new business product.
This helps companies assess a product's consumer response before deciding to apply it or not.This is the surest way for mangers to know the success potential of a new product or service. If J. C. Penney had done business experiment on Johnson’s change proposals, they would have noticed the negative consumer response.
Companies think that testing proposed innovations are expensive and time-consuming. This is the reason they feel reluctant to use it. Even so, despite the huge benefits of experimentation, it is not as simple as it sounds.
Running business experiments for a retail business involves many technical and organizational complexities.
It is easier to conduct test comparisons for internet-based businesses than conventional retail businesses. Conventional businesses have many store branches in several locations, which make business experiments difficult. Online tests give internet-based companies access to a large pool of consumers, from which they can do a random sampling of any number they want – even thousands. But this type of sampling is not possible with a conventional business. It is just impossible to carry out business experiments on thousands of store locations. This challenge usually causes a company to carry out tests on only a small number of its consumers, which is not enough to show a true reflection of the probable response of the majority of consumers. The result of such an incomprehensive test leads to managers making wrong decisions. To gain access to full information that will make the efforts of business experiment worthwhile, companies must first clarify certain points before the experiment.
These points are: - Purpose
- Commitment
- Reliability
- Feasibility
- Derivatives
Purpose
A business experiment must have a clear purpose. It must focus on a well-defined management initiative that is already under discussion. There must also be a clear-cut expectation of what people can learn from the experiment. Companies should use experiments as a practical tool for better understanding of executive initiatives. A case in point is that of big retail company Kohls.
In 2013, Kohl was seeking ways of lowering its cost of operation. 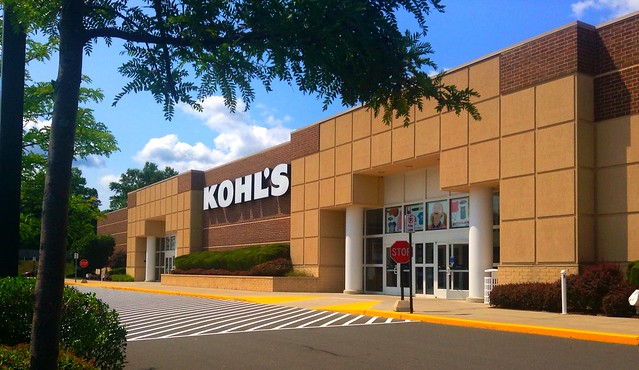
An idea suggested one-hour delay in opening of stores from Mondays to Saturdays. This threw up a strong debate within the company’s management.
One group supported the suggestion, while another claimed the action would reduce sales. The only way to know which group was right was to subject the idea to a comprehensive business experiment. After conducting a test across 100 stores, the result showed that a delay in store opening time would not have any serious negative impact on sales.
The purpose here was clear. Determine if the delayed opening of a store by an hour to reduce operation cost would cause a decline in sales.
CommitmentThe level of commitment of all the decision makers is another important point. All the stakeholders must agree to accept the experiment result. No sentiments should affect the execution of the result. All the team members must agree to accept the result in its entirety, and not consider just a part of it. If the result is not promising, they must all agree to abandon the idea.When Kohl's wanted to add furniture as a new product, the executives were optimistic. They thought the new product would have a favorable impact on the company's sales. Six months later, and with tests done at 70 stores, Kohl's was experiencing a decline in sales. The cause was that many of the company's products had to make way to create more floor space for the furniture. And this resulted in a decline in sales for those other products.
No matter how glorious the prospect seemed, Kohl's was losing both revenue and customers. All the stakeholders then agreed to scrap the idea.
Reliability
A business experiment will always produce a result. But, will the result be reliable? This pertinent question focuses on the method of experiment. Although defined procedures are available for carrying out any business experiment, companies usually compromise reliability with time, cost, and other practical elements. The following three methods of the experiment are useful for achieving result reliability.
Blind Tests - Blind tests help cut the manipulation of experiments - knowingly or unknowingly, by participants when they realize they are subjects of a business experiment. When participants are not aware they are under observation, they tend to behave more naturally, thus increasing the reliability of test results.
Random Trials - The idea behind randomization is straightforward - select a random group of subjects with common traits and separate them into a test group and a control group. Subject the test group to the experiment and check the reaction against the control group’s behavior. If the reaction is favorable, then the experiment result is reliable.
Big Data - As mentioned earlier, carrying out tests for online-based transactions involves a defined protocol. Yet, when dealing with physical businesses like retail stores, which have limited sample sizes for experiments, many variables should come into consideration. This is where big data comes in. For instance, assume a huge retail business that is considering remodeling its stores across a thousand locations at the cost of 500 million dollars. It remodeled 30 stores and monitored the results. The finance department did an analysis of the results and decided that an increase in sales because of the remodeling would be marginal, with no profit yield. The marketing department did its own analysis and projected a significant increase in sales. The finance department made use of only six months of data prior to and after the remodeling. It did not choose control stores from the same location as the test stores. In contrast, the marketing department used twelve months of data for its analysis. It also chose control stores within the same geographic location as the test stores. The company then used big data to determine the reliability of the two forecasts. Big data included more variables like weather, demographics, sales time, competition, prices etc. After applying appropriate statistical protocols, the retailer found out that the use of control stores in the same location as the test stores made the forecast by the marketing department more reliable.
Feasibility
How practical is the experiment? This is an important question for any company that is considering business experimentation. Companies must be able to test experiment forecasts. Test feasibility does not only rely on result comparison between a test group and a control group. It goes beyond that. In the current dynamic business environment, the likely causes of results are usually vague. There are complex relationships between variables, which are difficult to understand. This challenge makes it necessary to have a firm knowledge of a business environment. For an environment with a high level of causes, it is important to determine the feasibility of using a huge sample size to reduce the effects of various elements that are not under consideration. Using huge samples for tests is usually not cost-friendly and practical. Such data sizes can change operations dramatically. The better way would be to use a small sample size with big data analysis for a more a meaningful test result. Huge sample sizes do not naturally produce better results. A company does not need to sell directly to each of its customers to gain insight into consumer behavior. It can use a small number of distributors to collate and analyze consumer data. The size of a sample usually depends on the level of expected effect. A small sample size is perfect when the cause will have a big effect. But if the executives expect the cause to have a minimal effect, a huge sample size should apply. A high number of inferences is necessary to identify the expected effect from extraneous effects. The right sample size enables a company to save on testing costs and raises the level of innovation. It also ensures statistically correct test results.
Value Derivatives
Finally, it is worth knowing what the value derivatives from the business experiment are. Companies should determine not just what works, but more importantly, where they can work best. A good approach would be to determine the value created by the effect of testing an innovation in different segments, environments, and consumers. The focus should only be on areas where the largest value is achievable.
For instance, whenever Petco concludes a business experiment and the result is good, it does not just go ahead with a company-wide rollout.
It rolls out only in control stores that have common attributes with the test stores. With such focused rollout, Petco has saved the cost of rolling out to all stores. It has also avoided stores where the innovation may either not yield value. Petco's focused rollout has enabled it to double the forecasted value derivatives of its innovations.
CONCLUSION
Without proper analysis to find the cause of the results of a business experiment, companies will continue to make uninformed decisions. When Kohl's carried out a test on delayed opening of its stores, there was an initial decline in sales. If Kohl's had discontinued this initiative, it would have lost the potential benefits. A further analysis revealed that despite the drop in sales, the number of transactions per customer remained the same. The drop in sales was a reflection of an initial drop in the number of units per transaction. This later increased, driving up sales to its former levels and saving one-hour worth of operation cost. Management did not hurriedly equate the test results with the cause.
Companies are now realizing that experiment itself is just the first step. Value creation comes with an in-depth analysis of experiment results. Thus, business experiment enables executives develop better decision-making skills, through a comprehensive analysis of test results.